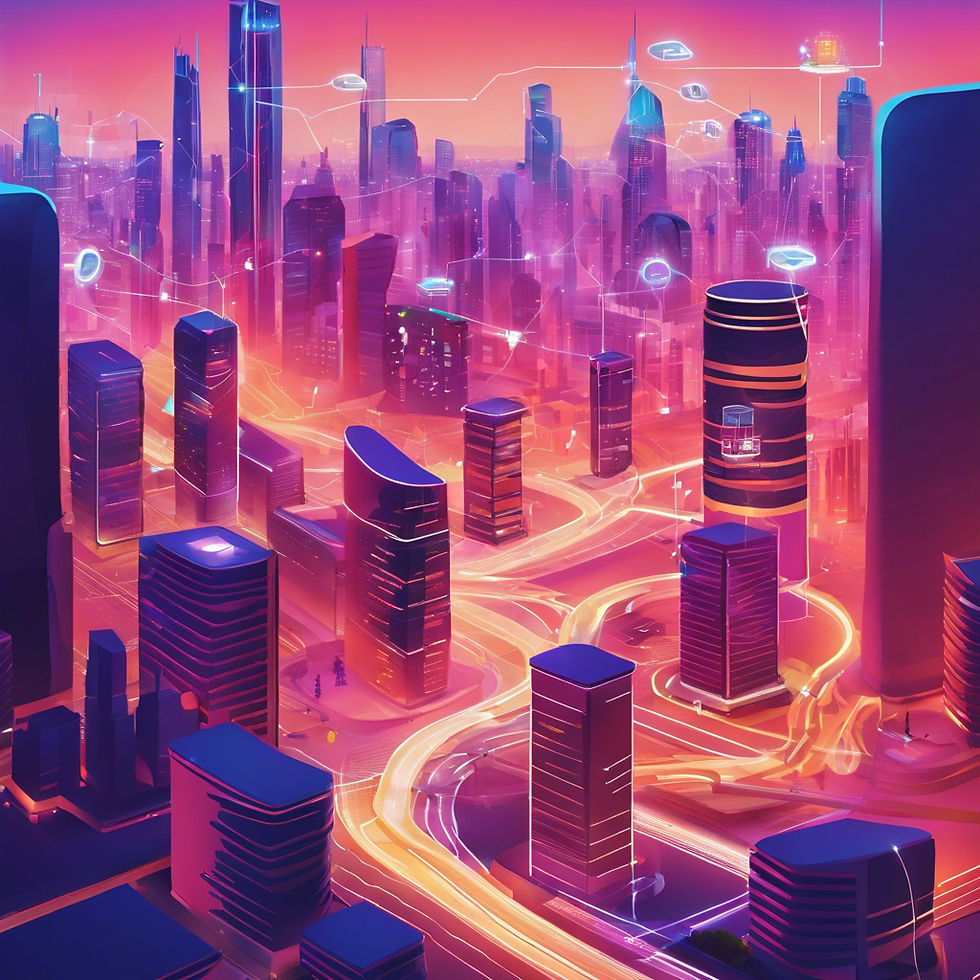
Edge computing is rapidly transforming the landscape of artificial intelligence (AI), particularly through the deployment of small mode
ls that operate directly on edge devices. This shift is crucial as it enables real-time data processing, reduces latency, and enhances privacy and security.
In this article, we will explore the implications of edge computing for AI, emphasizing how small models can lead to broader adoption of AI technologies.
The Rise of Edge Computing and AI
Edge computing refers to the practice of processing data closer to its source rather than relying on centralized cloud servers. This approach is becoming increasingly vital as organizations seek to minimize latency and improve user experiences. According to forecasts, global spending on edge computing is projected to reach $232 billion in 2024, reflecting a 15.4% increase from the previous year. The convergence of edge computing and AI—termed Edge AI—allows for the execution of machine learning models on local devices, which can lead to faster decision-making and reduced bandwidth usage.
Benefits of Edge AI
Reduced Latency: By processing data locally, edge AI can deliver real-time insights essential for applications such as autonomous vehicles and smart manufacturing.
Enhanced Privacy and Security: Sensitive data remains on the device rather than being transmitted to the cloud, thus minimizing exposure to potential breaches.
Lower Bandwidth Costs: Only processed metadata is sent to the cloud, significantly reducing the amount of data transmitted over networks.
Operational Efficiency: Edge AI supports autonomous operations, enabling systems to function effectively even in intermittent connectivity scenarios.
Small Models vs. Large Models
The debate between small models and large models in AI is pivotal for determining how organizations adopt these technologies:
Advantages of Small Models
Efficiency: Small models require less computational power, making them ideal for deployment on edge devices with limited resources.
Speed: They can process data quickly, which is critical for applications needing immediate responses.
Accessibility: Smaller models lower the barrier to entry for businesses looking to implement AI solutions without extensive infrastructure investments.
Challenges with Large Models
While large models, such as generative AI and large language models (LLMs), have garnered significant attention due to their capabilities, they often face practical limitations in edge environments:
Resource Intensive: Large models demand substantial computational resources and often require cloud-based infrastructure, which can introduce latency.
Complexity in Deployment: Implementing large models at the edge can be cumbersome and may not yield the necessary real-time insights that many industries require.
The Path Forward: General Adoption of AI
The thesis that small models will drive general adoption of AI is supported by several factors:
Increased Demand for Real-Time Processing: Industries such as healthcare, manufacturing, and smart cities are increasingly reliant on real-time data analysis. Small models are better suited for these environments due to their speed and efficiency.
Broader Accessibility: As small models become more prevalent, businesses without extensive technical expertise can leverage AI capabilities, democratizing access to advanced technologies.
Scalability: Organizations can deploy numerous small models across various devices rather than relying on a few large systems, enhancing flexibility and scalability.
Conclusion
As we move into 2025 and beyond, the integration of edge computing with AI will continue to evolve. The focus on small models presents a promising avenue for widespread adoption of AI technologies across diverse sectors. By enabling faster processing times, enhancing security measures, and lowering operational costs, small models are poised to play a critical role in shaping the future of artificial intelligence at the edge. Embracing this shift will be essential for businesses seeking to remain competitive in an increasingly digital landscape.